Understanding Titus Data Classification for Effective Management
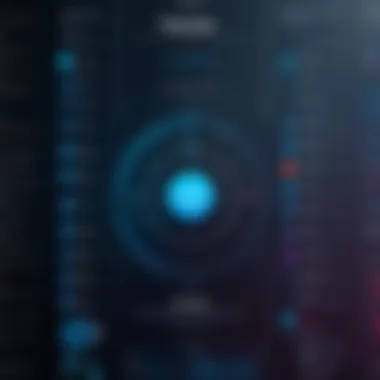
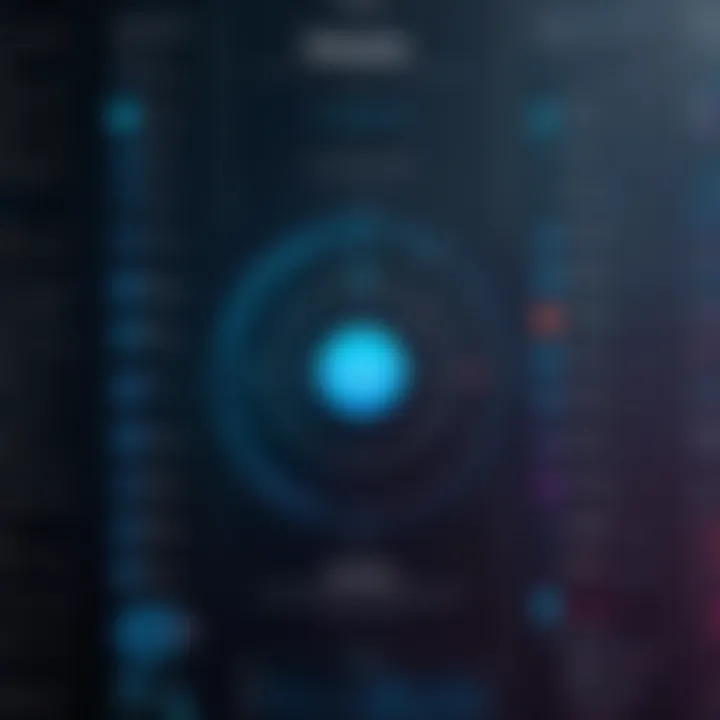
Intro
In the ever-evolving landscape of data management, understanding how to classify data efficiently is crucial. Titus Data Classification serves as a pivotal solution that enables organizations to safeguard, manage, and utilize their data. This process is not just about compliance; it is about enhancing operational effectiveness and organizational governance.
Titus offers various methods and technologies that cater to different sectors, facilitating decision-makers in making informed choices about data handling and protection. As data continues to proliferate, mastering classification techniques will be essential for IT professionals and entrepreneurs alike.
Software Overview
Brief Description of Software
Titus Data Classification is a sophisticated software solution designed to help organizations categorize their data accurately. By using predefined criteria and user-defined rules, it provides a structured approach to labeling sensitive information. This allows users to understand the importance of their data and take appropriate measures for its protection.
Key Features and Functionalities
Titus includes several key features:
- Data Discovery: Identifying sensitive data across various platforms and formats.
- Automated Classification: Utilizing machine learning to classify data automatically based on patterns.
- User-Defined Policies: Allowing administrators to set specific rules based on organizational needs.
- Reporting and Analytics: Providing insights into data classification trends and compliance requirements.
- Integration Capabilities: Seamlessly working with existing data systems and security solutions.
Importance of Data Classification
Data classification is more than just a tech buzzword. It plays a vital role in ensuring that sensitive data is protected appropriately. Neglecting proper classification can lead to data breaches and non-compliance with regulations. Thus, organizations must realize that classification should be a strategic initiative, not an afterthought.
Finale
In summary, Titus Data Classification represents an essential component for any organization looking to advance its data management strategies. As data continues to grow exponentially, adopting effective classification solutions will be a cornerstone of maintaining integrity and compliance. Understanding these practices will empower industry professionals to enhance their software advisory capabilities and improve their operational frameworks.
Prologue to Titus Data Classification
Data classification is a fundamental component of effective data management practices. In the context of Titus Data Classification, this concept translates into a robust framework designed to categorize information based on its sensitivity and importance. Appropriate data classification not only helps organizations in managing their data efficiently but also ensures compliance with various regulatory requirements. In todayโs data-driven environment, understanding how to implement these strategies through Titus is essential for all organizations.
Definition of Data Classification
Data classification can be defined as the process of organizing and categorizing data into specific groups based on defined criteria. This includes attributes such as sensitivity, relevance, and usage. By establishing these classifications, organizations can streamline their data handling processes, making it easier to access, manage, and safeguard data according to its level of sensitivity. Different categories can vary significantly, addressing public, internal, confidential, or restricted data.
Importance of Data Classification in Today's Landscape
In an era where data breaches and cyber threats are rampant, the significance of data classification cannot be overstated. Organizations are now compelled to comply with various legal frameworks, such as GDPR and HIPAA, which necessitate careful data handling practices.
Data classification supports this compliance by ensuring that sensitive information receives appropriate protection. By implementing a systematic approach to data categorization, organizations can minimize the risk of unauthorized access and potential liabilities. Furthermore, an effective classification strategy can enhance operational efficiency by facilitating better data management and retrieval practices.
"Data classification is not merely a regulatory requirement; it is a fundamental practice that enhances overall data governance and security."
In summary, proper data classification through Titus allows organizations to proactively address current challenges in data management, ensuring a secure and compliant operational landscape.
The Role of Titus in Data Management
In the world of data management, the role of Titus is becoming very crucial. Organizations are managing vast amounts of data. Ensuring the right data gets the right protection is necessary. Titus provides a framework that helps businesses categorize their data effectively. This classification is not only about security but also enables organizations to meet compliance requirements, optimize workflows, and support data governance initiatives. By using Titus, businesses can streamline their data management processes and minimize risks associated with data breaches or non-compliance.
Titus Overview
Titus is a software solution designed for data classification. It helps organizations identify, access, and manage their most valuable data assets. The software focuses on the systematic classification of information, making it easier for companies to segregate data based on sensitivity and importance. With a user-friendly interface, Titus allows IT teams to implement classification policies without extensive technical knowledge. Furthermore, its adaptability across various sectors means that it serves both small businesses and large enterprises effectively.
Key Features of Titus Data Classification Solutions
Titus is not just a data classification tool; it offers a range of features that enhance data management practices. Here are some key elements that make Titus stand out:
- Flexible Classification Models: Titus allows organizations to create tailored classification frameworks. This includes predefined categories that align with compliance standards like GDPR or HIPAA.
- Integration Capabilities: The software integrates seamlessly with various data management systems. This integration ensures that classification happens throughout the usual workflow without disrupting everyday tasks.
- User Engagement: Titus promotes user involvement in the classification process. Employees can classify data based on guidelines, ensuring that data handling is consistent across the organization.
- Reporting and Analytics: The platform provides robust reporting tools. Organizations can analyze data classification trends over time, allowing for better decision-making concerning data governance.
"Implementing effective data classification is not just about compliance; itโs about ensuring business continuity and data security."
Epilogue
These features and benefits show the necessity of Titus in todayโs data-driven environment. Organizations need strong classification tools to stay relevant and secure in their data handling practices.
The Framework of Data Classification
The Framework of Data Classification serves as a foundational element in the context of Titus Data Classification. This ensures effective management, protection, and governance of data. A well-structured framework directs organizations in categorizing their data based on specific criteria. This classification is essential primarily for compliance purposes and for protecting sensitive information from potential breaches. It also enhances data handling efficiency across departments.
Classification Categories
Public Data
Public Data includes information that an organization decides to make available to the general public. An example of Public Data is press releases, marketing content, or general operational summaries. The key characteristic of Public Data is its accessibility; it is intended to be viewed and utilized by anyone without restrictions.
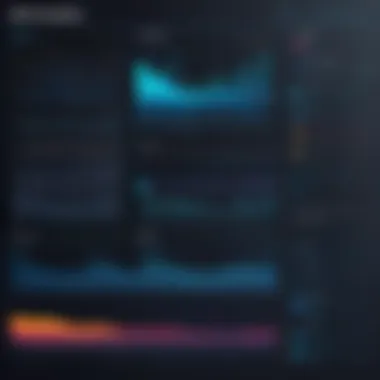
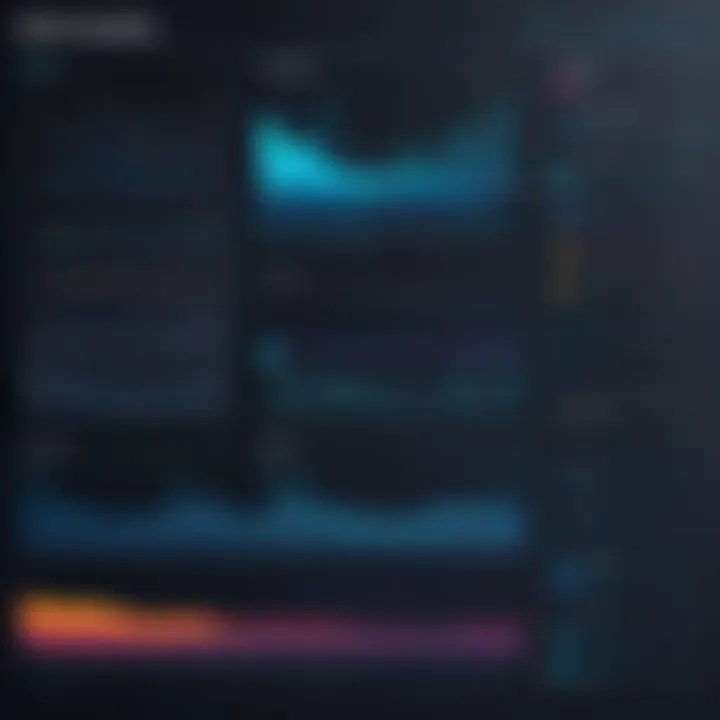
This classification is beneficial for fostering transparency and trust with stakeholders, as it enables open access to non-sensitive information. A unique feature of Public Data is its ability to enhance an organization's public image. However, it can be a disadvantage if sensitive internal information is unintentionally included.
Internal Use Only
Internal Use Only data is designed strictly for use within the organization. This may include internal reports, employee information, or training materials. The key characteristic here is that this data is not meant for public distribution and can contain proprietary insights.
It represents a popular choice for companies that wish to protect their competitive advantage. A unique feature of Internal Use Only data is the level of control it offers over information dissemination, but it may cause inefficiencies if access is too restricted, preventing collaboration.
Confidential Data
Confidential Data consists of sensitive information that requires significant protection due to legal or ethical considerations. Examples include financial records, customer data, and trade secrets. The key characteristic of Confidential Data is its restricted access; only authorized personnel can view it.
This classification is beneficial as it reduces risk and increases the trust of clients and stakeholders. A unique feature of Confidential Data is the need for regular security audits to ensure compliance with relevant regulations. However, managing Confidential Data can be resource-intensive due to these stringent security measures.
Restricted Access
Restricted Access data refers to information that has limited availability due to the potential harm its disclosure could cause. This may include classified government information or personal health records. The key characteristic is that access is highly regulated.
This classification is beneficial for ensuring that information is protected against unauthorized access and abuse. A unique feature of Restricted Access data is the need for a clear, enforceable policy regarding who can access this information. However, it presents challenges in efficiently sharing crucial information among relevant parties.
Data Sensitivity Levels and Their Implications
Data sensitivity levels categorize data based on how critical it is to an organization's operations and the potential consequences of its loss or exposure. Organizations must carefully assess these sensitivity levels to implement appropriate safeguards. This process is inherently linked to compliance and risk management.
Understanding the sensitivity levels of data assists in aligning data protection strategies with regulatory requirements.
By doing so, companies can ensure their data management practices support both business objectives and regulatory compliance. In this context, establishing a clear communication channel for all stakeholders is crucial for maintaining a secure data environment.
Titus Data Classification Methods
The methods of Titus Data Classification are crucial for ensuring effective data management and governance. Employing a combination of techniques allows organizations to categorize their data systematically. This categorization facilitates compliance with various regulations and also helps in protecting sensitive information. Understanding these methods can ultimately strengthen a companyโs overall data strategy.
Manual Classification Techniques
Manual classification involves the hand-picking of data for categorization. It is straightforward and allows for high precision, as data stewards can apply their knowledge. This method is often utilized when the data volume is less and when accuracy is paramount.
However, this approach can be time-consuming and labor-intensive. Some potential downsides include:
- Scalability Issues: As data increases, manual processes become burdensome.
- Human Error: Dependence on individuals can introduce mistakes in classification.
For organizations with lower data volumes or specific requirements, manual classification may still be the best fit. It fosters a thorough understanding of data and its contextual needs.
Automated Classification Processes
Automated classification has gained significance as data volume grows exponentially. This method leverages software tools that systematically classify data based on pre-defined rules and patterns. Automation reduces time spent on manual processes, facilitating efficiency and speed in handling large datasets.
Some key benefits of automated classification include:
- Increased Efficiency: Tasks that traditionally took hours can be reduced to minutes.
- Consistency: Automated systems apply the same rules uniformly, minimizing variations in classification.
- Scalability: It becomes easier to manage expansive data, which is critical for large enterprises.
Nonetheless, organizations should consider potential limitations, such as:
- Initial Setup Complexity: Developing rules and processes might require specialized knowledge.
- Contextual Understanding: Automated systems might miss nuances that a human could identify.
Machine Learning in Data Classification
Machine learning represents the frontier of data classification methods. This technique involves training algorithms to recognize patterns and make classifications automatically over time. Machine learning systems evolve and improve as they process more data, which significantly enhances data management.
Advantages of incorporating machine learning include:
- Adaptive Learning: Algorithms that learn and adjust classifications based on new data inputs.
- Higher Accuracy: With sufficient training, machine learning can surpass human classifiers in consistency and reliability.
- Predictive Analysis: Beyond simple classification, these systems can provide insights and forecasts about data usage and management.
However, organizations should keep in mind:
- Data Quality: Quality of input data is crucial for the performance of machine learning models.
- Resource Intensive: Initial investment in technology and expertise can be significant.
By combining manual, automated, and machine learning classification methods, organizations can build a robust framework for data classification and governance. Each method has its own strengths and can be applied based on specific needs and circumstances.
Implementing Titus Data Classification
Implementing Titus Data Classification is crucial for organizations aiming to enhance their data management strategies. Proper implementation of this classification can lead to improved data governance, risk management, and compliance with regulatory standards. By systematizing data classification, companies can ensure that sensitive information is adequately protected while remaining accessible for those who need it. The benefits of implementing these practices include increased efficiency, reduced data breaches, and better alignment with organizational policies.
Preparing for Implementation
Preparing for implementation starts with a foundational understanding of current practices and future needs. This section outlines two essential steps: Assessing Current Data Management Practices and Identifying Stakeholder Needs.
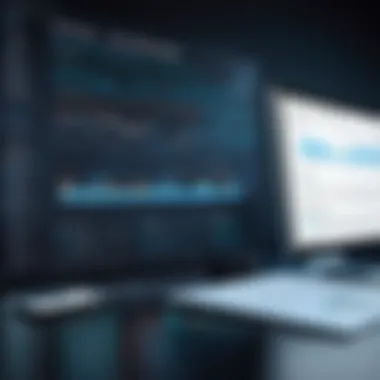
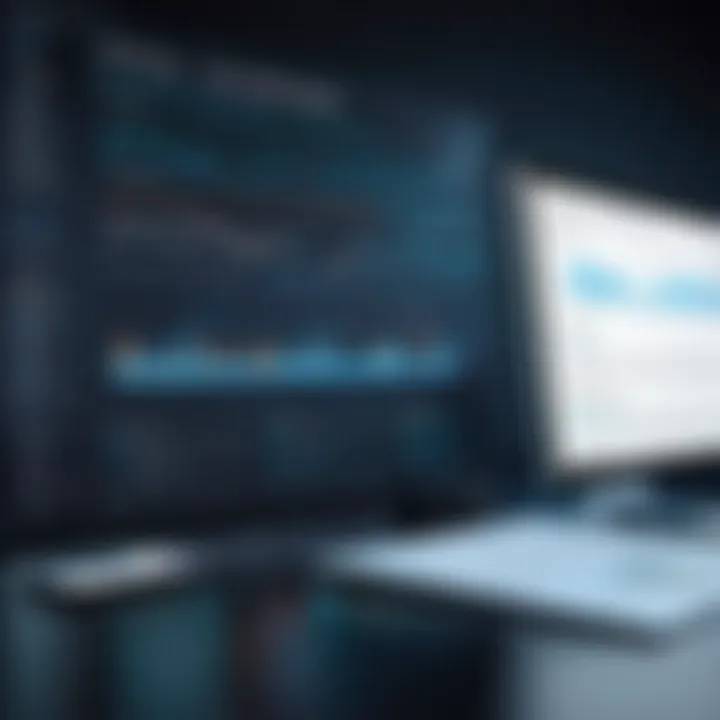
Assessing Current Data Management Practices
Assessing current data management practices involves a thorough review of existing systems and processes. This assessment helps to identify weaknesses, gaps, and areas for improvement. One key characteristic of this assessment is that it brings to light how data is currently classified and handled within the organization. It is beneficial because it establishes a baseline from which enhancements can be made.
Notably, the unique feature of this assessment is its focus on operational workflows. It allows organizations to pinpoint inefficiencies that may lead to data mishandling or non-compliance. The advantages of this approach are clear; by understanding these practices, data classification can be streamlined and aligned with best practices.
Identifying Stakeholder Needs
Identifying stakeholder needs is vital for successful implementation of data classification. This involves engaging with all parties that interact with data, including IT teams, compliance officers, and end-users. One key aspect here is understanding the specific requirements and concerns of these stakeholders regarding data access and protection.
The identification process is a popular choice because it ensures that the implementation is collaborative and takes into account different perspectives. A unique feature of this step is the inclusion of feedback mechanisms; this allows adjustments to be made to the classification framework based on stakeholder input. Advantages of focusing on stakeholder needs include increased buy-in and more effective classification strategies that ultimately benefit the entire organization.
Establishing a Data Classification Policy
Establishing a data classification policy is a significant step in the implementation process. A well-defined policy serves as a guiding framework for how data should be classified, handled, and protected. This policy must take into account various factors including regulatory requirements, risk analysis, and industry standards. By creating clear guidelines, organizations can ensure consistent data handling practices across all departments, leading to enhanced compliance and security.
Training and Support for Staff
Training and support for staff is critical in ensuring the success of Titus Data Classification implementation. All employees should be educated on their roles in maintaining data integrity and security. A robust training program should encompass the classification policy, data handling techniques, and the importance of compliance. Providing ongoing support through resources, such as workshops or a dedicated help desk, encourages staff engagement and reinforces learning.
The foundation of successful data classification lies not only in technical measures but in a well-informed and committed workforce.
With these steps in place, organizations can build a solid framework for implementing Titus Data Classification, leading to meaningful improvements in data governance and security.
Challenges in Data Classification
Data classification plays a pivotal role in data management and compliance. However, it is not without its challenges. Organizations often encounter various obstacles that can hinder effective data classification. Understanding these challenges is essential for developing strategies to mitigate them, ensuring that data remains secure and compliant with regulations.
Data Classification Complexity
One of the primary challenges in data classification is the inherent complexity involved in categorizing vast amounts of data. Organizations produce an overwhelming quantity of data daily. This deluge can make it difficult to accurately classify each data piece. Furthermore, the data comes from diverse sources, including emails, documents, databases, and cloud storage. This variety can lead to inconsistencies in classification practices, as different data types may require distinct approaches.
The complexity is exacerbated by the ever-evolving regulatory landscape, which mandates stricter compliance measures. As regulations change, organizations must adjust their classification strategies accordingly. This can create confusion among staff who may not be fully trained to implement new guidelines or understand the implications of misclassification. The result can be a fragmented data classification framework that risks compliance violations and data security breaches.
Resistance to Change within Organizations
Another significant challenge is the resistance to change that often exists within organizations. Employees may be accustomed to existing processes and may view new data classification protocols as an inconvenience. This resistance can stem from various factors, including fear of the unknown, perceived increased workload, or a lack of understanding regarding the importance of data classification.
For successful implementation of data classification, it is crucial to foster a culture of acceptance around these changes. Organizations must communicate the benefits of data classification to all team members. This can include protecting sensitive information and enhancing overall data governance. Training programs should be established to educate employees on the importance of compliance and data protection. In doing so, organizations can reduce resistance and empower staff to embrace new practices.
"Organizations must prioritize change management to address resistance, ensuring that all employees understand their roles in data classification processes."
Navigating the challenges of data classification is essential for organizations striving to maintain data integrity and compliance. By addressing complexity and resistance to change, businesses can create a more robust classification framework that supports consistent data governance.
Compliance and Regulatory Considerations
In the realm of data management, compliance and regulatory considerations are paramount. Organizations increasingly face pressures to adhere to various data protection laws and regulations. This necessity to comply not only affects how businesses handle data but also influences their overall operational strategies.
Titus Data Classification plays a vital role in achieving compliance with regulatory requirements. By categorizing sensitive data appropriately, organizations can enforce specific access controls, ensuring that the right individuals have the correct level of access to classified materials. This system of categorization also helps mitigate risks associated with misuse or unauthorized access.
Furthermore, compliance serves as a framework within which organizations can build trust with clients. A demonstrated commitment to regulatory standards can establish a solid reputation, which translates to customer loyalty. Conversely, neglecting compliance can lead to severe consequences, including legal action and damage to an organization's credibility.
Data Protection Regulations
Data protection regulations are diverse and vary by region. For instance, the General Data Protection Regulation (GDPR) in the European Union is stringent, imposing robust requirements on data handling practices. Similarly, the California Consumer Privacy Act (CCPA) has set a high standard for how businesses manage consumer data in the United States.
Adherence to such regulations necessitates active data classification efforts. Organizations must ensure they identify and protect personally identifiable information (PII), confidential business information, and other sensitive data as defined by these laws. Failure to systematically classify this data can lead to potential breaches of data protection regulations, resulting in costly penalties.
Moreover, updates to these regulations often require organizations to adapt their data classification strategies. Keeping abreast of legislative changes is crucial for ensuring sustained compliance.
Impact of Non-Compliance
The consequences of non-compliance with data protection regulations can be severe. Monetary penalties may be the most apparent repercussion, but the fallout can extend beyond fines. Non-compliance often results in reputational damage that could dissuade potential customers. In many cases, consumers are keen to do business with organizations they trust to protect their information.
In addition to financial penalties and loss of reputation, organizations may also face operational disruptions. Investigations stemming from breaches can lead to resource allocation challenges, diverting attention from core operations and stalling business initiatives.
"Non-compliance with regulations is not merely a legal burden; it extends to a reputational risk that organizations cannot afford to ignore."
The implications of neglecting compliance highlight the criticality of Titus Data Classification. By implementing a robust classification system, organizations design proactive measures that foster compliance and reduce potential adverse outcomes.
Integration with Other Systems
Integrating Titus Data Classification with other systems is essential for a comprehensive approach to data management. This integration supports seamless data flow across platforms while enhancing data security and compliance strategies. Here are a few specific elements and benefits to consider:
- Enhanced Data Security: By collaborating with existing security solutions, organizations can ensure that sensitive data remains protected according to classification guidelines. This synergy helps prevent data breaches and unauthorized access.
- Streamlined Data Management: Interfacing with data management systems simplifies tasks like data retrieval, processing, and reporting. Such integrations make it easier to manage large volumes of data effectively.
- Improved Compliance: Regulatory requirements often mandate specific data handling and protection measures. Integrating Titus with compliance-focused tools can help organizations adhere to regulations consistently and avoid penalties.
- Operational Efficiency: Real-time data flow and automated processes reduce manual intervention. This improves productivity and allows teams to focus on core tasks rather than low-level data management issues.
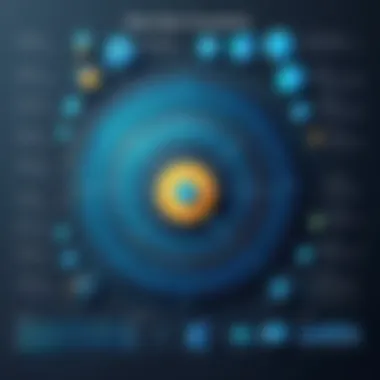
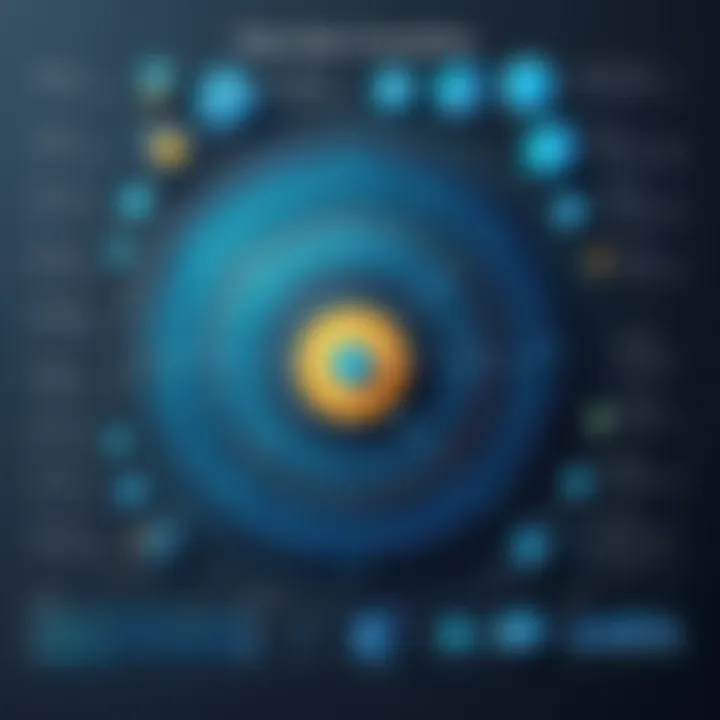
This chapter explores two key aspects of this integration crucial in today's data-driven landscape.
Collaboration with Security Solutions
Collaboration between Titus and security solutions is vital for maintaining data integrity. When Titus operates effectively alongside platforms like Symantec, McAfee, and Cisco, the risks associated with data classification significantly diminish.
- Centralized Control: Security solutions provide a centralized means to manage data access and rights based on classification levels. This ensures that employees access only the information necessary for their roles, enhancing privacy and security.
- Threat Detection: Integrating with security systems can aid in proactive threat detection. This means organizations can identify potential vulnerabilities in their data classification processes before they become significant issues.
Moreover, using advanced security measures can prevent data leaks when handling classified information. This partnership can extend to implementing protective measures for sensitive data both at rest and in transit.
Interfacing with Data Management Systems
Interfacing Titus with data management systems streamlines operations in organizations while enhancing data governance and efficiency. Popular platforms like Microsoft SQL Server and Oracle Database often serve as primary data repositories.
- Data Lifecycle Management: This integration facilitates better data lifecycle management by automatically classifying data as it moves through different stages. This capability ensures that older data is archived or deleted according to compliance requirements.
- User-Friendly Access: When users access data classified through Titus on established data management systems, the experience is seamless. Clear visibility into classification ensures employees can easily locate the information they need.
- Analytics and Reporting: By connecting classification efforts with data analytics tools, companies can derive insights based on data sensitivity. This approach allows organizations to identify potential risks and areas of improvement in their data strategy.
In summary, the integration of Titus Data Classification with existing systems is not just advisable but essential. By fostering strong connections with security solutions and data management platforms, organizations can optimize their data governance efforts and ensure compliance in a complex regulatory landscape.
Evaluating Titus Data Classification Success
Evaluating the success of Titus Data Classification is a crucial step for organizations looking to optimize their data management practices. Understanding how well these data classification methods are performing can highlight the effectiveness of the implemented strategies. Success in data classification not only supports compliance with regulations but also enhances data governance and security. This evaluation process stems from clear goals and employs specific metrics to measure outcomes.
Key Performance Indicators (KPIs)
Key Performance Indicators, or KPIs, serve as measurable values that demonstrate how effectively an organization is achieving key business objectives. For Titus Data Classification, relevant KPIs can include:
- Accuracy of Classification: This measures how often data is classified correctly. A higher accuracy rate indicates better performance of the classification system.
- User Adoption Rate: This reflects how many users are actively utilizing the data classification tools within the organization. Increased adoption suggests successful integration into workflow.
- Time Saved in Data Retrieval: This assesses the efficiency gained in locating and managing data. A decrease in retrieval time can be a significant benefit of effective classification.
- Compliance Rate: Regular assessments can show compliance with relevant regulations. A higher rate symbolizes successful adherence to established guidelines.
- Incident Reduction: Tracking data breaches or losses before and after implementing Titus data classification can provide insight into its effectiveness in protecting sensitive information.
Evaluating these KPIs allows organizations to fine-tune their data classification processes and address any shortcomings in a proactive manner.
Continuous Improvement Strategies
Continuous improvement is fundamental to sustaining success in any data management system, including Titus Data Classification. It involves regularly assessing the classification methods and implementing strategies that focus on enhancements. Here are some strategies for promoting continuous improvement:
- Regular Training: Ongoing training for staff ensures they stay informed about new features and updates in Titus. Educated users are essential for maintaining data accuracy and security.
- Feedback Mechanisms: Establishing a system for gathering user feedback helps identify challenges faced by staff during data classification. This input can inform adjustments in strategies or tools.
- Benchmarking Against Industry Standards: Comparing classification efforts to industry benchmarks provides valuable insights. This practice can reveal areas for improvement and best practices worth adopting.
- Automated Auditing: Regular audits of the classification process using automated tools can pinpoint areas needing enhancement without the resource-intensive nature of manual checks.
- Updating Policies: As regulations evolve, updating classification policies to reflect changes in compliance requirements is necessary. Reviewing policies regularly ensures that they remain relevant and effective.
Future Trends in Data Classification
Data classification is evolving rapidly due to technological advances and shifting regulatory landscapes. Organizations must keep pace with these changes to ensure robust data governance. Future trends will shape how data classification tools are designed and implemented, directly impacting data management success and compliance.
Emerging Technologies
Emerging technologies play a vital role in the future of data classification. Innovations like artificial intelligence, machine learning, and advanced analytics enhance classification processes. For instance, artificial intelligence can automate the classification of vast data sets. This reduces reliance on manual inputs, which are often error-prone and time-consuming. Furthermore, machine learning algorithms improve over time by analyzing patterns in data, enhancing accuracy in classification.
Additionally, blockchain technology offers secure data traceability. With blockchain, organizations can track how data is categorized and modified, ensuring that sensitive information remains protected.
Key benefits of these technologies include:
- Increased accuracy: Automation reduces human error.
- Cost efficiency: Streamlined processes lower operational costs.
- Enhanced scalability: Organizations can manage larger data volumes easily.
Changing Regulatory Requirements
As data protection regulations continue to evolve, organizations must adapt their data classification strategies accordingly. New laws often demand stringent classification standards and practices. This affects how data is managed and shared, particularly for sensitive information.
For instance, the General Data Protection Regulation (GDPR) in Europe has significantly impacted data classification norms globally. Organizations are increasingly required to classify personal data to ensure compliance with privacy laws. Failure to adhere can result in severe penalties. Therefore, it is crucial for businesses to stay informed about regulatory changes and adjust their classification policies accordingly.
Important considerations for organizations include:
- Understanding regional regulations: Knowing laws in different regions helps in compliant data management.
- Regular audits: Continuous assessment of classification methods ensures alignment with the law.
"Organizations that prioritize compliance adapt better to regulation changes and gain a competitive edge".
By proactively addressing these emerging trends and changes in regulations, organizations can foster an environment of effective data classification and ensure compliance while driving innovation.
Closure
The conclusion of this article holds significant value as it encapsulates the main themes presented throughout the discussion on Titus Data Classification. In todayโs data-driven world, effective data classification is not just a technical requirement but a strategic necessity for organizations, ensuring sensitive data is managed properly while complying with regulatory obligations.
Summarizing Key Insights
In summarizing the key insights, we can reflect on some essential points:
- Importance of Data Classification: Proper classification of data enhances protection. It helps organizations identify and manage data risks effectively. This is crucial in maintaining compliance and achieving overall business security.
- Titus Functionality: The versatility and robustness of Titus as a data classification solution are key to navigating complex data environments. The platform enables organizations to implement a systematic classification strategy, improving data governance and reducing security breaches.
- Methods and Frameworks: Various methods such as manual, automated, and machine learning techniques offer scalability. These methods allow organizations to classify large volumes of data efficiently, ensuring proper categorization based on sensitivity and usage.
- Implementation Strategies: The importance of having a well-defined data classification policy cannot be overstated. Engaging with key stakeholders during implementation increases the likelihood of successful integration and adoption.
- Future Outlook: Finally, recognizing future trends in data classification is essential. Keeping an eye on emerging technologies and changing regulatory requirements will assist organizations in refining their classification strategies, better preparing them for forthcoming challenges.
Call to Action for Industry Professionals
Industry professionals are encouraged to take a proactive approach to data classification. Here are some actionable steps to consider:
- Assess Current Practices: Regularly evaluate your existing data management strategies. Ensure they align with best practices in data classification and comply with relevant regulations.
- Leverage Technology: Utilize advanced tools and technologies like Titus to enhance your data classification capabilities. This can bolster security and improve operational efficiencies.
- Continual Learning: Stay informed about trends and regulatory changes affecting data classification. This knowledge can guide necessary adjustments in policies and practices.
- Promote a Culture of Data Awareness: Encourage all stakeholders to understand the importance of data classification within your organization. This may require training and resources, but improving data awareness is vital to effective governance.
Titus Data Classification presents various opportunities for transforming organizational data management. By actively engaging in the classification process, professionals can better protect their data assets, meet compliance standards, and develop a more secure digital environment.