Essential Software Solutions for Data Analysis
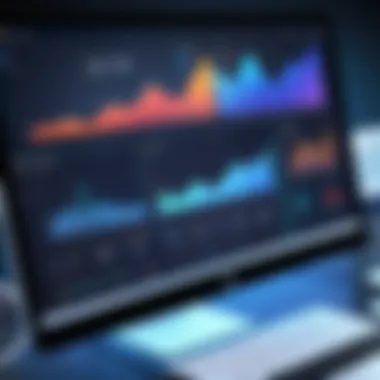
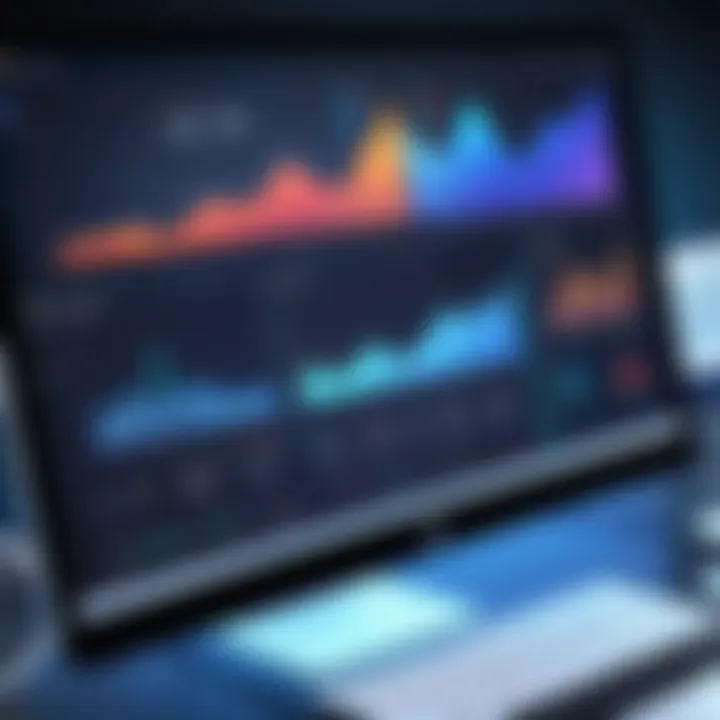
Intro
In the current age, vast amounts of data are generated every second. Businesses, researchers, and organizations across various industries recognize the growing importance of data analysis software. These tools facilitate insightful decision-making, improve operational efficiency, and provide a competitive edge in an increasingly complex market landscape. This overview aims to guide industry professionals in understanding the diverse software options available, their core features, and how to select the right one for their needs.
Software Overview
Brief Description of Software
Data analysis software is designed to help users interpret complex data sets, transforming raw numbers into meaningful insights. Various applications range from statistical analysis and business intelligence to machine learning and predictive analytics. Each software solution varies in complexity, functionality, and user interface. Therefore, selecting the right tool involves understanding specific project requirements and user proficiency.
Key Features and Functionalities
When exploring software for data analysis, several critical features stand out:
- Data Integration: The ability to connect with various data sources, such as databases, spreadsheets, and APIs, is essential.
- Statistical Analysis Tools: These features allow users to perform a range of statistical operations, from basic calculations to complex modeling.
- Visualization: Effective data visualization aids in understanding data trends and insights. Options like graphs, charts, and dashboards are valuable.
- Collaboration Tools: Many software solutions offer collaboration features that enable teams to work together seamlessly.
- Automation Capabilities: Users benefit from tools that automate repetitive tasks, allowing them to focus on high-value activities.
"Choosing the right data analysis software can greatly affect the success of a project and influence overall business strategies."
Detailed Comparison
Comparison with Competitors
Not all data analysis software is created equal. A detailed comparative analysis can shed light on how different tools measure up against each other. Some notable software solutions in the market include:
- Tableau: Renowned for its robust visualization capabilities, Tableau provides advanced analytics and user-friendly dashboards.
- Microsoft Power BI: Offers extensive integration with other Microsoft products, making it a convenient option for businesses already using the ecosystem.
- R and Python: While not conventional software solutions, these programming languages offer extensive libraries for statistical analysis and data manipulation.
Each product has its strengths and weaknesses, particularly in ease of use, integration capabilities, and scope of functionalities. Evaluating these elements is crucial for a well-informed decision.
Pricing Structure
Understanding the pricing structures for data analysis software is also vital. The costs can vary significantly:
- Subscription-based Billing: Many tools like Microsoft Power BI and Tableau operate on a subscription basis, charging monthly or annually.
- One-time Licensing: Certain options, such as SPSS, require an upfront payment, followed by periodic upgrade fees.
- Open Source Solutions: Software like R and Python are free to use, which can be appealing for budget-conscious users. However, they may require a steeper learning curve.
Understanding Data Analysis Software
Data analysis software is fundamental in today's business environment. Its relevance extends across industries, as organizations strive to turn raw data into actionable insights. Understanding this software encompasses recognizing its definition, purpose, and core components. This exploration allows decision-makers and IT professionals to select tools that align with their specific needs. Evaluating these aspects not only leads to better-informed choices but also enhances overall data-driven strategies.
Definition and Purpose
Data analysis software refers to tools designed to help users collect, organize, analyze, and visualize data. The primary purpose of these applications is to extract value from vast amounts of information. Such software can aid in identifying trends, patterns, and relationships that may not be immediately obvious. Users can conduct statistical analyses or create visual representations of data to facilitate decision-making. Whether it's for market research, financial forecasting, or operational optimization, the software's definition and purpose underscore its significance in contemporary data practices.
Importance in Modern Business
In modern business, the ability to analyze data effectively is a competitive advantage. Companies are inundated with data from various sources, including customer interactions, sales transactions, and social media. Data analysis software enables organizations to harness this information, transforming it into strategic insights.
The importance lies in the following aspects:
- Enhanced Decision-Making: Accurate analyses lead to informed choices, improving responses to market changes.
- Operational Efficiency: By analyzing workflow and performance data, businesses can identify inefficiencies, leading to cost reductions.
- Customer Insights: Understanding customer behavior through data analysis assists in tailoring services and products.
"Data is the new oil. Itโs valuable, but if unrefined it cannot really be used."
โ Clive Humby
Key Components of Effective Data Analysis
When evaluating data analysis software, several key components must be considered:
- Data Collection: Effective software should facilitate easy data import from various sources, ensuring data integrity.
- Analytical Tools: The presence of statistical tools, machine learning algorithms, and data manipulation capabilities is crucial.
- Visualization Features: Good software provides robust visualization options, allowing users to present data findings in a clear, comprehensible manner.
- User Interface: A well-designed interface enhances usability, making it accessible for both technical and non-technical users.
Each of these components plays a vital role in establishing an effective data analysis environment. By understanding these elements, organizations can maximize the advantages offered by such software, fostering a culture of informed decision-making.
Types of Data Analysis Software
Understanding the different types of data analysis software is crucial for businesses seeking to leverage data effectively. Each category serves unique purposes, aligning with specific analytical needs. This section delves into three primary types of data analysis software: Descriptive Analytics Software, Predictive Analytics Tools, and Prescriptive Analytics Solutions. By recognizing their distinctions, decision-makers can better evaluate which tools suit their analytical objectives.
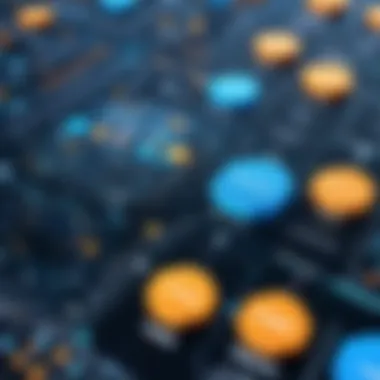
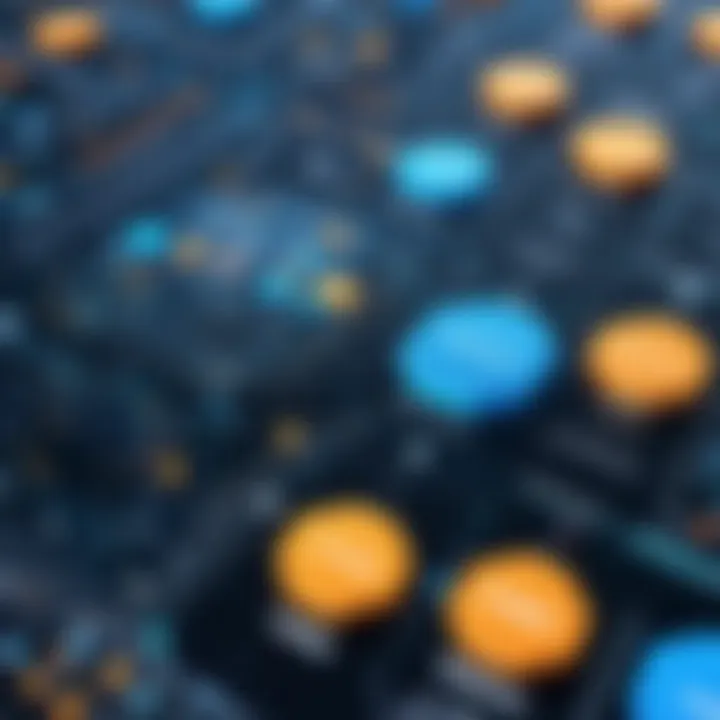
Descriptive Analytics Software
Descriptive analytics software provides insights by summarizing historical data. It answers the question of what has happened. Common tools in this category include Tableau and Microsoft Power BI. These platforms utilize past data to generate reports, dashboards, and visualizations. The emphasis here is on data aggregation and presentation, helping organizations understand trends over time.
Key Benefits:
- Data Summarization: Making complex data more digestible.
- Trend Analysis: Identifying long-term patterns.
- Visualization Tools: Enhancing comprehension through graphics.
Decision-makers often depend on descriptive analytics to make sense of operational performance. For example, a retail company may utilize descriptive analytics to analyze sales data from the previous quarter, providing insights into inventory levels and customer purchasing behavior.
Predictive Analytics Tools
Predictive analytics takes analysis a step further by using historical data to forecast future outcomes. This type of software employs statistical models and machine learning techniques. Notable examples include IBM SPSS Statistics and SAS Analytics.
These tools allow organizations to make informed predictions, such as customer churn rates or sales forecasts. By understanding potential future scenarios, businesses can allocate resources strategically and adjust marketing efforts.
Considerations:
- Data Quality: Reliable predictions depend on clean data.
- Model Accuracy: Continuous evaluation is necessary to optimize predictions.
Predictive tools are integral in industries such as finance, where firms use them for credit scoring and risk assessment. An accurate forecast helps to mitigate financial risks and improve overall decision-making.
Prescriptive Analytics Solutions
Prescriptive analytics goes beyond prediction; it recommends actions based on data analysis. This category includes tools that suggest optimal strategies to achieve specific objectives. Solutions like Python libraries often play a vital role in developing custom algorithms for unique business challenges.
Advantages:
- Actionable Insights: Directing users on optimal choices.
- Resource Efficiency: Identifying the best allocation of resources.
- Scenario Simulation: Testing various scenarios to find the best outcomes.
Businesses employing prescriptive analytics can navigate complex environments with confidence. For instance, a manufacturing company might utilize prescriptive solutions to optimize supply chain operations. The ability to simulate changes and predict outcomes informs smarter choices.
Prescriptive analytics empowers organizations to not only predict outcomes but also to make informed decisions based on those predictions.
In summary, data analysis software is not a one-size-fits-all approach. Each type serves distinct needs and offers unique benefits. Understanding these facets is critical for professionals making software decisions, enabling them to deploy the right tools for their specific analytical goals.
Key Features to Consider
When evaluating software for analyzing data, it is critical to identify specific features that align with organizational goals. These features not only enhance user experience but also determine the softwareโs overall effectiveness in processing and interpreting data. The right software can lead to better business decisions and more efficient data management. In this section, we will explore several essential features that every useful data analysis software should possess.
Ease of Use and Interface Design
A straightforward interface is essential for data analysis software. Users typically seek tools that allow for quick navigation and efficient workflow. Intuitive design minimizes the learning curve, enabling users to focus on analysis rather than struggling with software complexity. A user-friendly dashboard can significantly impact how effectively teams interact with data.
Many modern tools prioritize usability by incorporating drag-and-drop functionalities and clear menu options. This design facilitates seamless data import, exploration, and visualization. It is worth considering software that provides extensive help resources, such as tutorials and community forums to further assist users in overcoming software hurdles.
Integration Capabilities with Other Systems
Integration with other systems is another key feature. Data does not exist in isolation; therefore, the ability to connect with various databases, cloud services, and applications is vital. Software capable of integrating with existing business tools can streamline processes.
For instance, connecting data analysis software with platforms like Salesforce or Microsoft Excel can enhance productivity and data accuracy. This capability not only reduces redundancy but also ensures that users access real-time data during their analysis. When reviewing options, assess the types of integrations offered and how they align with your organization's current technology stack.
Visualization and Reporting Tools
Strong visualization capabilities are a necessity for data analysis software. Users should be able to create clear and informative charts, graphs, and dashboards. Visualization transforms raw data into understandable formats, facilitating better communication of insights across teams.
Moreover, reporting tools that allow customization can significantly enhance the interpretation and presentation of findings. These reports should be easily shareable across the organization. Organizations often benefit from software that provides automated reporting features, reducing the time needed to generate insights and freeing resources for deeper analysis.
Scalability and Customization Options
Scalability is particularly significant for growing organizations. The chosen software should adequately support future needs without requiring a complete overhaul. This means looking for tools that can handle increasing volumes of data and users without sacrificing performance.
Additionally, customization options are key in tailoring the software to meet specific organizational needs. Some organizations may require advanced statistical functions, while others might focus on user access levels and data security measures. Flexible software solutions provide options to adapt features and functionalities according to evolving business requirements.
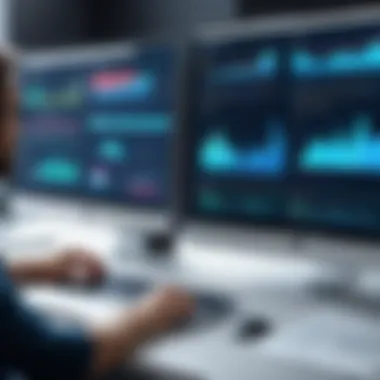
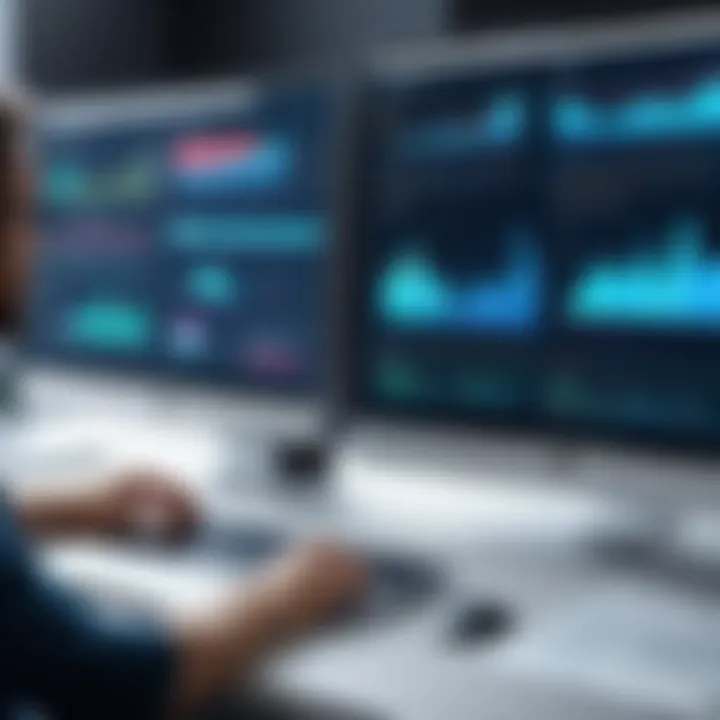
"Choosing the right data analysis software requires careful consideration of various features that directly influence usability and effectiveness."
Top Software Solutions for Data Analysis
The selection of the right software solution for data analysis is crucial for organizations seeking to leverage their data effectively. This section aims to provide insights into prominent software options available today, emphasizing their unique capabilities and benefits. Understanding these tools allows decision-makers to choose solutions that align with their specific analytical needs and business objectives.
Tableau
Tableau stands out as a leading player in the market for data visualization and analysis. Its user-friendly interface allows for intuitive drag-and-drop functionalities, making it accessible to users with different levels of technical expertise. With the ability to connect to various data sources, Tableau facilitates the creation of interactive dashboards and visualizations that can simplify complex data narratives.
One of Tableau's strengths lies in its powerful analytics capabilities. It enables users to perform ad hoc analyses, uncover trends, and extract meaningful insights from data sets effortlessly. Teams can collaborate in real time, sharing insights and visualizations seamlessly, which enhances decision-making processes across the organization.
Microsoft Power BI
Microsoft Power BI represents a robust business analytics solution that provides detailed visualizations and business intelligence capabilities. Power BI integrates seamlessly with other Microsoft services, making it a natural choice for organizations already utilizing tools like Excel or Azure. Its dashboarding features allow users to create visually rich reports that summarize key metrics at a glance.
Power BI not only simplifies data discovery but also supports data preparation and transformation. Users can model their datasets, apply advanced analytics functions, and even share insights across various platforms securely. Furthermore, the affordability combined with its comprehensive feature set makes Power BI an attractive option for companies looking to scale their data analytics efforts.
IBM SPSS Statistics
IBM SPSS Statistics is a powerful software suite known widely for its statistical analysis capabilities. It is particularly favored in academic settings and industries where robust statistical methodologies are crucial. Users can perform complex statistical tests easily, generate reports, and visualize outcomes with clarity.
The software is designed to cater to data scientists and analysts who require sophisticated predictive analytics and hypothesis testing. Its ability to handle large datasets efficiently allows for advanced modeling and forecasting, which can directly influence strategic decisions. Organizations invested in research and data-heavy industries will find significant value in SPSS Statistics as it helps in deriving insights from vast amounts of data.
R and Python for Data Analysis
R and Python are programming languages that have gained substantial traction within the data analysis community. They offer flexibility and a wide range of libraries tailored for various analytics tasks. R is particularly strong in statistical analysis and visualization, while Python is renowned for its versatility and ease of use.
Utilizing R or Python for data analysis can facilitate custom solutions, advanced analytics, and machine learning applications. The open-source nature of these languages allows organizations to leverage community-driven innovations continually. Furthermore, potential users benefit from extensive documentation and resources available online.
SAS Analytics
SAS Analytics has long been recognized as a leader in advanced analytics, business intelligence, and data management. The software provides a comprehensive suite of tools designed for statistical analysis, predictive modeling, and data visualization.
SAS is particularly notable for its strong data governance and security features, making it ideal for industries with strict compliance requirements. Its extensive analytical capabilities find applications in sectors ranging from finance to healthcare. Users appreciate the depth of analytics offered, as well as the ability to scale solutions to meet growing data demands. SAS Analytics empowers organizations to make data-driven decisions that are both timely and informed.
Evaluation Criteria for Selection
When selecting data analysis software, decision-makers must consider various evaluation criteria. This section emphasizes the significance of a systematic approach to identifying the right tool. The process not only impacts the efficiency of data analysis but also defines the potential return on investment. A well-structured evaluation process helps organizations avoid costs associated with software that does not meet their specific needs.
Assessing Cost-Effectiveness
One of the primary concerns for any organization is cost-effectiveness. Evaluating the overall cost of a software solution includes initial expenses such as licensing fees, implementation costs, and training. However, looking solely at initial costs can be misleading. It is essential to assess long-term value, including ongoing support, updates, and scalability. A cost-effective solution should save time and resources while delivering accurate insights.
Factors to consider include:
- License Types: Different software may have varied licensing structures, impacting overall costs. Organizations should explore options like subscription models versus one-time purchases.
- Hidden Costs: Often, additional costs arise in the forms of support or integration fees. Clear transparency from vendors about these costs is crucial.
- Quantifiable Benefits: Determining potential gains from the software through improved decision-making is vital. Products that enhance efficiency can lead to significant financial returns over time.
Understanding Licensing Models
Another critical aspect of selecting data analysis software is understanding its licensing models. The flexibility and constraints of a licensing model significantly influence how organizations operate and scale their data initiatives. Each model comes with unique advantages and drawbacks.
- Enterprise Licensing Agreements: Suitable for larger organizations, these agreements often provide greater savings but can be complex. They can also restrict usage across departments unless properly managed.
- User-Based Licensing: Ideal for smaller teams or businesses, this model often enables easy scaling as organizations grow. However, it might become costly with an increasing number of users.
- Open Source Licenses: These options may reduce financial barriers but often require technical expertise to implement effectively. Organizations must consider whether they have the necessary resources for maintaining and customizing the software.
User Reviews and Case Studies
User feedback and empirical evidence through case studies offer invaluable insights during the selection process. Critical analysis of user experiences helps to uncover strengths and weaknesses that may not appear in marketing materials. Organizations should seek reviews across various platforms to gain a comprehensive view of software performance.
- Online Reviews: Platforms like Reddit and product comparison websites can provide an unfiltered look at user experiences. Feedback trends may highlight persistent issues or outstanding features of the software.
- Case Studies: Reading about how similar industries have implemented solutions can reveal real-world applications. Case studies often provide clear images of ROI and the software's impact on business processes.
Evaluating data analysis software through these lenses ensures organizations can identify the best tool tailored to their unique requirements. By focusing on cost-effectiveness, understanding licensing, and leveraging user experiences, decision-makers can make informed choices that foster success in their data initiatives.
Emerging Trends in Data Analysis Software
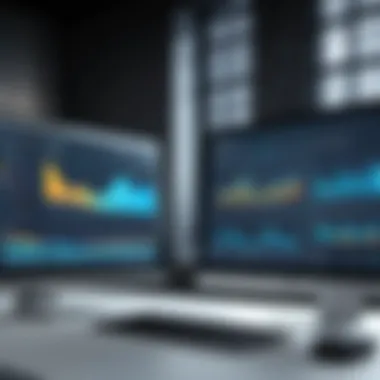
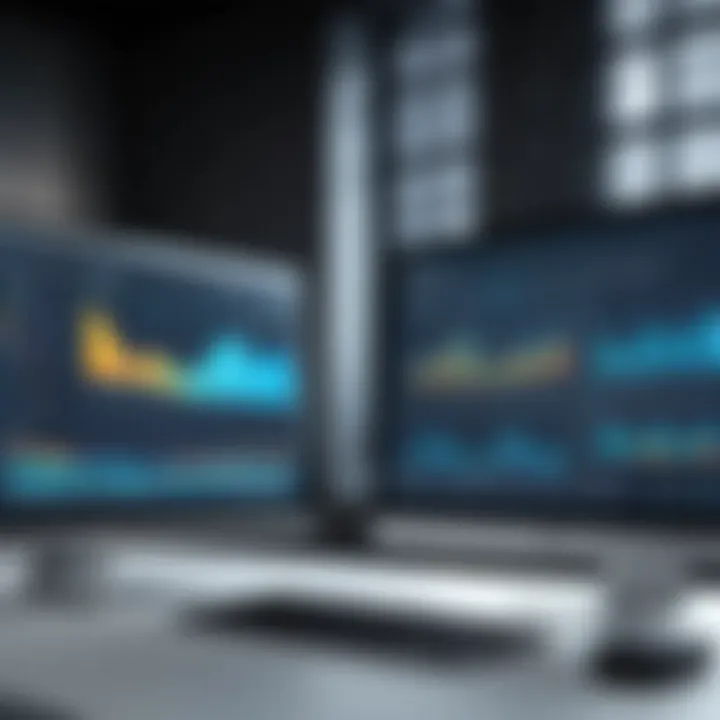
The landscape of data analysis software is in a constant state of flux. Emerging trends shape how businesses and organizations interact with data. By understanding these elements, decision-makers are better equipped to embrace innovations that can enhance their analytical capabilities. The integration of cutting-edge technologies is crucial for staying competitive in todayโs data-driven world.
Artificial Intelligence and Machine Learning Integration
Artificial intelligence and machine learning are at the forefront of transforming data analysis software. These technologies enable systems to learn and adapt without explicit programming. They provide advanced predictive analytics, which can identify patterns and trends that humans might overlook. This leads to more accurate forecasts and better decision-making. Data-driven insights generated from AI models can directly influence strategy and operational efficiency.
Moreover, organizations are encouraged to consider how AI can improve data quality. Automated data cleaning and preparation are essential to ensure reliable outputs. As a result, tools that integrate AI not only speed up analysis but also enhance consistency and reliability in results. The increased sophistication of these tools makes them pivotal in any serious data strategy.
Cloud-Based Solutions
Cloud computing has revolutionized the way data analysis software is offered and utilized. Organizations increasingly move to cloud-based platforms due to their flexibility, scalability, and cost-effectiveness. These solutions eliminate the need for extensive on-premises installations and maintenance. Instead, users can access powerful tools directly via the internet, which reduces the overhead associated with traditional software management.
In addition, cloud-based systems foster collaboration, allowing teams to work on the same data simultaneously from different locations. This accessibility is paramount for enterprises with remote or dispersed teams. Businesses can now leverage extensive computing power and storage capacity with minimal upfront investment.
Furthermore, data security in cloud environments is continuously improving, which mitigates concerns related to sensitive information. Cloud providers are investing heavily in encryption and compliance measures, making it a trustworthy choice for businesses of all sizes.
Real-Time Data Processing Capabilities
The ability to process data in real-time is becoming increasingly important. Organizations require instant insights to react swiftly to dynamic business environments. Real-time data processing capabilities equip decision-makers with up-to-date information, enabling them to respond effectively to emerging trends and challenges.
For instance, industries such as finance and retail benefit greatly from real-time analytics. They can analyze consumer behavior and market movements as they happen. This allows for immediate adjustments to marketing strategies, operational procedures, or inventory management. Furthermore, the demand for real-time data is pushing software developers to innovate their platforms continually.
Common Challenges Faced by Users
Understanding the challenges that users encounter while utilizing data analysis software is crucial for making optimal software selections. Many professionals, including decision-makers in businesses and IT specialists, often face hurdles that can impede effective data analysis.
This section elucidates those common challenges, focusing on data quality and consistency issues, complexity in implementation, and the delicate balance needed between analysis and decision making.
Data Quality and Consistency Issues
One of the primary concerns users face in data analysis is ensuring data quality and consistency. Poor data quality can lead to erroneous conclusions and misguided strategies. Complications arise when data originates from multiple sources with varying formats. Inconsistent data not only creates confusion but also adds substantial time to the analysis process.
To mitigate these issues, users need to implement robust data-cleaning protocols. More importantly, they should prioritize standardization of data collection methods and establish clear guidelines for data entry. Regular audits of the data can help identify quality issues early, allowing teams to amend any inaccuracies. Utilizing software tools that offer data validation features can also significantly improve consistency.
Complexity in Implementation
Another significant challenge is the complexity involved in implementing data analysis software. Many solutions available are not intuitive, requiring extensive training or technical knowledge. Without a proper understanding of the software, users can struggle to utilize its features effectively, resulting in underutilization of the investment.
To smoothen the transition, businesses should consider adopting user-friendly software that offers seamless onboarding experiences. Workshops and continuous training can equip team members with necessary skills. Additionally, selecting software with strong customer support can aid in addressing implementation hurdles. Having access to tutorials and documentation can further simplify the learning curve for new users.
Balancing Analysis with Decision Making
Finally, there is the challenge of balancing detailed data analysis and timely decision-making. In fast-paced environments, an in-depth analysis might delay critical business decisions. Conversely, quick decisions based on incomplete data can lead to negative outcomes. Finding the right balance requires effective communication and organization within teams.
Encouraging cross-functional collaborations can ensure that those who analyze data work closely with decision-makers, leading to more effective insights. Leveraging visualization tools can facilitate clearer presentations of data, thus helping stakeholders grasp essential insights quickly. Often, utilizing software that allows for rapid data interpretation can support prompt decision-making while still considering the quality of analysis.
"The effectiveness of data analysis software often lies in how well users can navigate its complexities and apply findings in real-world scenarios."
Future of Data Analysis Software
The landscape of data analysis software is evolving rapidly. As organizations generate more data than ever, the importance of effective tools for analyzing this information cannot be overstated. The future of data analysis software holds significant promise, with advancements that will enhance how businesses leverage their data. Understanding these trends is crucial for decision-makers, IT professionals, and entrepreneurs, as it will influence their strategies and technology investments moving forward.
Evolution of User Interfaces
User interfaces have come a long way from the early days of data analysis software. They are becoming increasingly intuitive, allowing users of varying skill levels to engage with complex data sets. The shift towards more interactive designs emphasizes visual storytelling. This includes drag-and-drop functionalities and customizable dashboards. These changes minimize the learning curve for users who are not data specialists, fostering a culture where data-driven decision-making is accessible.
In the future, we may see augmented reality (AR) tools that provide real-time insights through immersive and interactive visualizations. This evolution will enable organizations to analyze and interpret data in ways that were previously unimaginable. User interfaces will also likely incorporate voice-activated commands or AI-driven suggestions to expedite the analysis process, further simplifying user experience.
Increased Accessibility for Non-Technical Users
One key trend is the increased focus on making data analysis software user-friendly for non-technical users. The barriers that once limited access to data analysis tools are slowly being dismantled. This means that even those without a formal background in data science can utilize analytical tools effectively. By simplifying functionalities and making training resources more readily available, software developers are broadening their user base.
Accessibility is not merely about the software itself but also about the support provided. Tutorials, community forums, and comprehensive documentation are becoming standard offerings. This democratizes data analysis, allowing more individuals to contribute insights and drive decisions within their organizations. As accessibility increases, so does the potential for innovation and creativity in utilizing data across sectors.
Growth of Community-Based Support
As data analysis software continues to advance, there is a notable trend towards community-based support structures. These communities allow users to share knowledge, troubleshoot issues, and discuss best practices. Such platforms foster collaboration among users who may face similar challenges.
The growth of these communities can be observed on platforms like Reddit, where discussions around software usage and trends in data analysis thrive. Communities offer significant advantages. Users can receive feedback from peers and experts alike, improving their understanding of the tools available to them. This peer-reviewed approach to learning can enhance problem-solving efficiency and lead to better outcomes in data analysis.