Big Data Analytics Software: A Comprehensive Overview
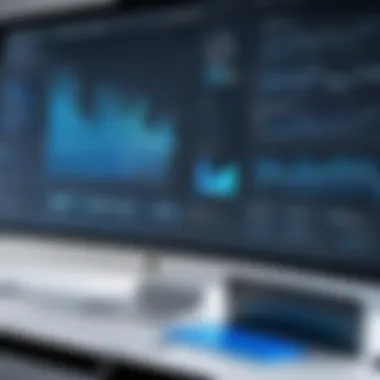
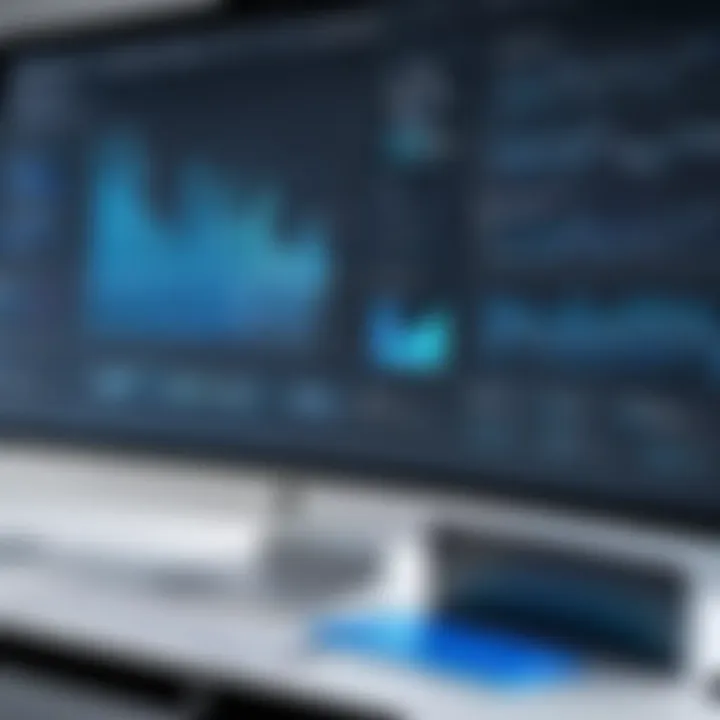
Intro
In the landscape of modern business, big data analytics software has emerged as a pivotal tool in shaping strategies and driving efficiencies. This software enables organizations to harness vast amounts of data, transforming raw information into actionable insights. Its relevance in today’s decision-making processes cannot be overstated, as businesses increasingly rely on data-driven approaches to remain competitive and relevant in their industries.
The evolution of big data analytics is intertwined with advancements in technology and changing consumer behaviors. Companies are seeking to not just collect data, but to analyze it thoughtfully and effectively. With a focus on functionalities and benefits, this article delves deeply into the intricacies of big data analytics software, exploring how it can support growth and innovation across various sectors.
Software Overview
Brief Description of Software
Big data analytics software encompasses tools and platforms designed to process and analyze large volumes of data at high velocity. These software solutions help organizations identify patterns, trends, and correlations within complex datasets. Popular examples include Microsoft Azure, Tableau, and Apache Hadoop, each offering unique capabilities tailored to meet the diverse needs of businesses.
Key Features and Functionalities
The core functionalities of big data analytics software often encompass several key features:
- Data Integration: Seamlessly combines information from various sources, ensuring comprehensive analysis.
- Real-time Analytics: Allows for immediate insights, enabling organizations to respond quickly to changes in the marketplace.
- Visualization Tools: Facilitates the representation of data in a visually intuitive format, making it easier for stakeholders to grasp complex information.
- Machine Learning: Integrates predictive analytics capabilities to forecast outcomes based on historical data.
- Scalability: Adapts to growing data volumes without significant performance degradation.
These features contribute to the enhancement of overall business performance by empowering users to make informed decisions that are both timely and strategic.
Detailed Comparison
Comparison with Competitors
When considering big data analytics software, it’s crucial to evaluate how different solutions stack up against each other. For instance, while Microsoft Azure provides strong integration capabilities with other Microsoft services, Tableau offers superior visualization options that can be more attractive for users who prioritize data presentation. Apache Hadoop excels in processing vast amounts of data affordably but may require a steeper learning curve compared to user-friendly interfaces like those offered by Qlik.
Pricing Structure
The pricing for big data analytics software varies significantly based on features and deployment options. Microsoft Azure operates on a pay-as-you-go model, which can be beneficial for businesses looking to minimize initial investments. In contrast, Tableau offers subscription plans that scale with the number of users and required features, providing flexibility for growing organizations. Review of competitive pricing can provide insights into the best investment for specific business needs, considering budget constraints and desired functionalities.
"Understanding the complexities of big data analytics software not only aids in selecting the right tools but also empowers organizations to leverage data for strategic advantage."
As the data landscape evolves, organizations must remain informed about the capabilities and offerings of available software. This awareness aids in navigating challenges and maximizing potential gains.
Prolusion to Big Data Analytics
The introduction of big data analytics marks a pivotal shift in how organizations interpret vast amounts of information. This shift is crucial for decision-makers, IT professionals, and entrepreneurs who seek to harness the potential of data in a rapidly evolving digital landscape. The emergence of big data analytics software has revolutionized data processing, enabling organizations to extract meaningful insights from complex datasets.
Defining Big Data
Big data refers to datasets that are so large or complex that traditional data processing software is inadequate to handle them. It spans three dimensions: volume, velocity, and variety. Volume indicates the sheer quantity of data generated every second, while velocity addresses the speed at which this data is created and processed. Variety refers to the different types of data formats available, such as structured, unstructured, and semi-structured data.
The importance of defining big data lies in identifying its characteristics to better manage and analyze it. Organizations must adopt appropriate technologies that can handle these complexities. As they navigate this terrain, understanding what constitutes big data becomes essential for effective data governance and strategy formulation.
Importance of Big Data Analytics
The significance of big data analytics cannot be overstated. It provides organizations with various benefits, such as enhanced decision-making, improved operational efficiency, and targeted marketing strategies. Big data analytics enables firms to discover patterns and trends that might not be visible through traditional analysis. The ability to analyze real-time data helps manufacturers optimize production processes and improve supply chain management.
Moreover, big data analytics supports risk management by predicting potential market disruptions and identifying strategies to mitigate them. The healthcare sector has also witnessed its utility, where predictive models analyze patient data to improve care delivery.
Adopting big data analytics is no longer a luxury but a necessity. Organizations that leverage it can gain a competitive advantage through informed decisions and strategic insights, leading to sustained growth and superior market positioning.
"In the age of information, understanding big data is key to unlocking business potential and fostering innovation."
Key Features of Big Data Analytics Software
Understanding the key features of big data analytics software is essential for decision-makers and IT professionals. This knowledge helps in identifying tools that meet specific organizational needs, optimize operational efficiency, and enhance overall business intelligence.
There are several key features that distinguish effective big data analytics software. Each feature plays a significant role in maximizing the potential derived from data-driven insights. Here, we will discuss three critical aspects: data processing capabilities, visual analytics, and predictive analytics.
Data Processing Capabilities
Data processing capabilities form the backbone of any big data analytics software. Such software must efficiently capture, store, and analyze vast amounts of data from diverse sources.
- Scalability: A well-designed processing framework can handle increasing amounts of data. This ensures the software remains useful as the volume of data grows.
- Speed: Real-time or near real-time data processing is essential for organizations that rely on timely insights. Long processing times can hinder decision-making processes.
- Versatility: The ability to process structured and unstructured data enhances the software's functionality. Organizations generate various data types, and the tools should adapt accordingly.
Investing in software with strong data processing capabilities allows businesses to derive value from their data efficiently. This ultimately leads to better decision-making across departments.
Visual Analytics
Visual analytics is an increasingly significant feature. This aspect transforms complex data sets into understandable visual formats, aiding in the comprehension of trends and patterns.
- Intuitive Dashboards: Intuitive dashboards allow users to quickly view key performance indicators (KPIs). This means decision-makers can assess data without delving into technical details.
- Interactive Graphics: Software that offers interactive graphs and charts enables users to explore data dynamically. This feature enhances engagement and understanding.
- Data Storytelling: Combining visual elements with data narratives can improve the communication of insights. Users can convey findings more effectively to stakeholders through visuals.
In a landscape where data visualization can significantly impact decision processes, investing in visual analytics capabilities is crucial for organizations.
Predictive Analytics
Predictive analytics represents an advanced capability that combines statistics, machine learning, and data mining. This feature analyzes current and historical data to forecast future outcomes.
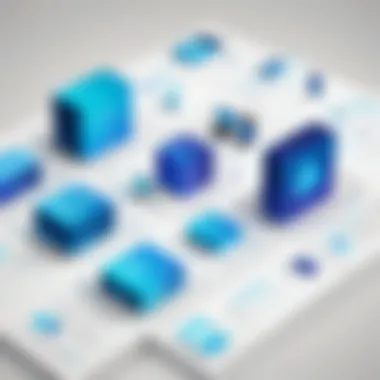
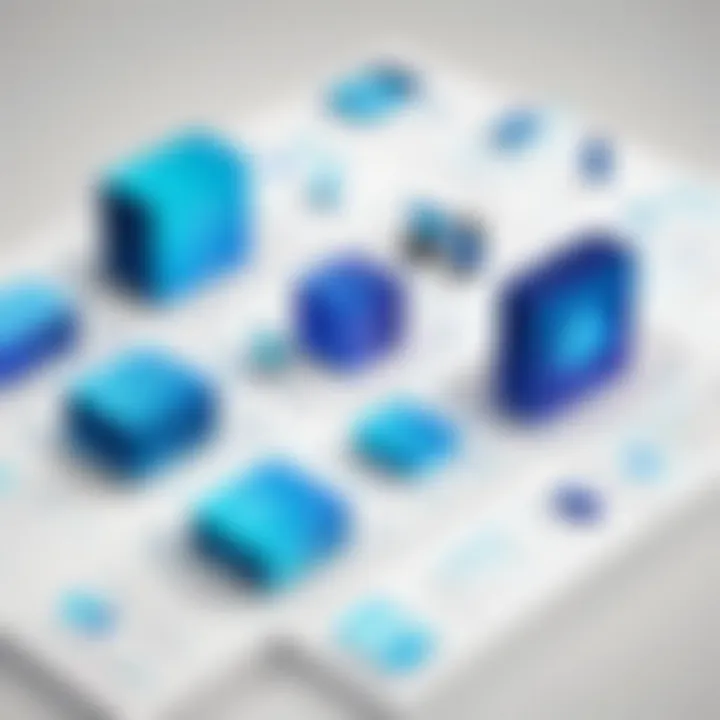
- Forecasting Trends: Predictive analytics tools aid in identifying trends before they become evident. This proactive approach can lead to strategic advantages in competitive environments.
- Risk Management: Organizations can assess potential risks by examining historical patterns. This proactive analysis enables better planning and decision-making.
- Customer Insights: By analyzing consumer behavior, companies can anticipate needs and preferences. This information is vital for marketing and product development.
The integration of predictive analytics into software is essential, as organizations increasingly rely on data-driven insights for strategic planning.
In summary, the key features of big data analytics software — data processing capabilities, visual analytics, and predictive analytics — play pivotal roles in shaping an organization’s data strategy. Choosing software that excels in these areas is fundamental for successful data utilization.
Deployment Models for Big Data Analytics
Deployment models play a crucial role in big data analytics. They determine how organizations can harness data effectively depending on their needs and resources. Understanding these models can help organizations make informed decisions about infrastructure, scalability, and costs.
On-Premises Solutions
On-premises solutions involve installing big data analytics software directly onto a company's servers. This model gives organizations full control over their data and infrastructure. Companies often prefer this approach for several reasons:
- Data Security: Sensitive data stays within company premises, minimizing risks associated with external breaches.
- Customization: Organizations can tailor analytics tools to their specific needs and workflows.
- Compliance: Some industries require stringent compliance measures. On-premises systems allow for greater oversight.
However, the on-premises model has drawbacks. Companies face significant upfront costs. They need to invest in hardware and the expertise to manage it. Additionally, maintenance and software updates can strain internal resources.
Cloud-Based Analytics
Cloud-based analytics is increasingly popular among organizations. This model allows companies to access analytics tools and data processing capabilities via the Internet. The benefits of cloud-based solutions include:
- Scalability: Organizations can easily adjust resources based on demand without heavy investments.
- Cost-Effectiveness: There are lower upfront costs. Companies typically operate on a pay-as-you-go basis, which can be financially advantageous.
- Accessibility: Analytics tools can be accessed from anywhere. This enhances collaboration among teams who work remotely or in different locations.
Nevertheless, organizations must consider potential issues, such as data privacy and dependence on Internet connectivity. Despite these challenges, cloud-based analytics is transforming how businesses operate.
Hybrid Models
Hybrid models combine on-premises and cloud solutions, allowing organizations to enjoy the benefits of both environments. This approach is often seen as a balanced way to manage data effectively. Key advantages include:
- Flexibility: Organizations can keep sensitive data in-house while using cloud services for less-sensitive information.
- Business Continuity: In case of disruptions in one environment, operations can continue in the other.
- Cost Management: Organizations can allocate resources efficiently, reducing potential expenditures.
Although hybrid models offer numerous benefits, managing a diverse infrastructure can complicate operations. Organizations must ensure compatibility between systems and maintain effective integration strategies.
"The right deployment model hinges on an organization’s unique needs, industry requirements, and future growth plans."
Selecting the Right Big Data Analytics Software
Choosing the appropriate big data analytics software is a pivotal decision for any organization. The vast amounts of data generated daily require robust tools for analysis to derive meaningful insights. Selecting the right software can enhance decision-making and operational efficiency. Businesses must understand their unique requirements, budget constraints, and the reputation of software providers when navigating this complex landscape.
Assessing Business Needs
Before committing to any analytics solution, organizations should perform a thorough assessment of their business necessities. Identifying the specific goals and objectives of big data initiatives is essential. For instance, does the company aim to improve customer segmentation, enhance operational efficiency, or perhaps drive innovations in product development?
Moreover, consider the volume and variety of data your organization deals with. Knowing whether the data is structured, semi-structured, or unstructured greatly influences the software choice. The analytics tool must align with these parameters to ensure effective data processing. Conducting stakeholder interviews and holding workshops with relevant teams can also uncover the hidden needs of the organization.
Budget Considerations
Budgeting for big data analytics software cannot be overlooked. Organizations often face a broad spectrum of options, each with its own pricing structure. It is crucial to examine not just the initial costs but also ongoing expenses such as subscription fees and maintenance costs.
Setting a budget helps narrow down choices significantly.
- Free and Open-Source Options: Tools like Apache Hadoop are powerful yet free to use. They can be economical for startups with limited funds.
- Commercial Solutions: These offer support, training, and advanced features. Microsoft Power BI and Tableau come at a cost but also provide comprehensive user experiences.
It is advisable to weigh the expenses against potential ROI from implementing these tools, ensuring that the software selected will deliver value over time.
Vendor Reputation
Vendor reputation plays a significant role in the selection process. A well-respected vendor often indicates reliability, quality of support, and product maturity. Researching vendors helps decision-makers assess their standing in the market.
- User Reviews and Case Studies: Look for testimonials and success stories. These provide insight into real-world applications of the software.
- Industry Recognition: Awards and recognitions can also indicate a vendor’s commitment to quality and innovation.
- Support Services: Evaluate customer support offerings. A vendor that provides robust support can enhance user experience, especially during implementation.
Making an informed choice involves extensive research. The right vendor will align software capabilities with business needs, ensuring successful adoption and usage.
Popular Big Data Analytics Tools in the Market
Big data analytics tools play a crucial role in helping organizations manage and extract valuable insights from vast data sets. These tools facilitate the processing, analysis, and visualization of big data, allowing businesses to make data-driven decisions. In this article section, we will delve into some of the most prominent tools in the market today, showcasing their unique capabilities and benefits.
Apache Hadoop
Apache Hadoop is an open-source framework designed for the distributed storage and processing of large data sets. It utilizes a system of clusters to allow organizations to store vast amounts of data across multiple machines, enhancing scalability and fault tolerance. One of Hadoop's strengths is its ability to handle various data formats, making it versatile in processing unstructured data, such as text, images, and audio.
The Hadoop ecosystem includes several key components:
- Hadoop Distributed File System (HDFS): This allows data to be stored across multiple nodes, ensuring redundancy and accessibility.
- MapReduce: A programming model for processing data in parallel across the cluster.
- YARN: A resource management layer that helps in job scheduling and resource allocation.
Hadoop's flexibility and cost-effectiveness make it a popular choice among businesses looking to harness the power of big data.
Apache Spark
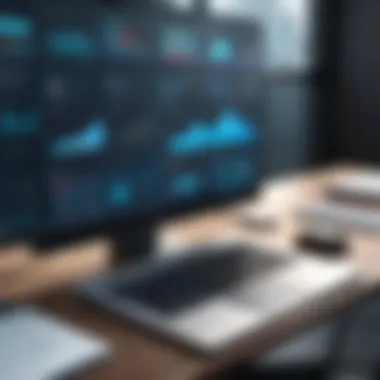
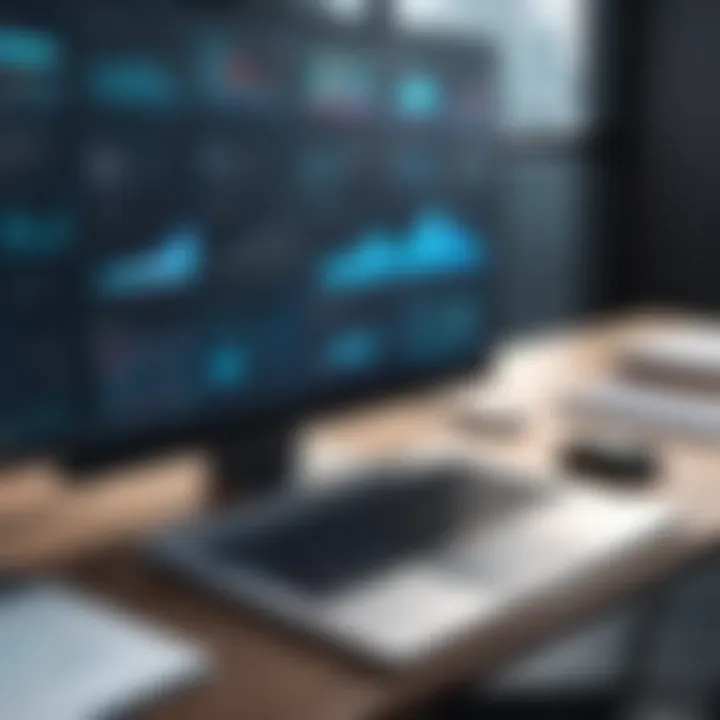
Apache Spark is another open-source framework that focuses on speed and ease of use. It is designed to provide fast, in-memory data processing, which significantly improves performance compared to disk-based processing solutions. Spark supports various programming languages, including Java, Scala, Python, and R, making it accessible to a wider audience of developers and data scientists.
Key features of Apache Spark include:
- In-memory Computing: This allows data to be processed much faster than traditional systems.
- Advanced Analytics: Spark supports machine learning, stream processing, and graph processing, addressing a wide range of analytical needs.
- Unified Engine: Users can run batch processing, real-time analytics, and machine learning workflows using a single platform.
Due to its high performance and versatility, Apache Spark has become a top choice for organizations aiming to perform complex data analysis quickly.
Tableau
Tableau is a powerful data visualization tool that enables users to create interactive and shareable dashboards. Its user-friendly interface allows for easy data exploration and visualization without requiring extensive programming knowledge. Tableau connects to various data sources, including databases and cloud services, facilitating real-time data analysis.
Notable features of Tableau include:
- Drag-and-Drop Interface: This simplifies the process of building visualizations.
- Collaboration Capabilities: Users can easily share dashboards and insights across teams, promoting a collaborative environment.
- Support for Big Data: Tableau can connect to big data sources directly, making it a great companion to tools like Hadoop and Spark.
Tableau's emphasis on visual storytelling makes it suitable for decision-makers who need to quickly comprehend complex data trends.
Microsoft Power BI
Microsoft Power BI is a suite of business analytics tools aimed at visualizing data and sharing insights across an organization. It enables users to create reports and dashboards that are insightful and visually appealing. Power BI integrates seamlessly with other Microsoft products, such as Excel and Azure, thus enhancing its usability for businesses already using Microsoft's suite.
Key benefits of Microsoft Power BI include:
- Real-Time Data Access: It allows continuous monitoring and analysis of business metrics.
- Customizable Reports: Users can design tailored reports to meet specific business needs.
- Integration with Azure: This enables the processing and visualization of big data directly from Azure services, enhancing performance.
Power BI has gained popularity for its accessibility and integration capabilities, making it an essential tool for modern businesses looking to harness big data analytics.
"The choice of analytics tool can significantly impact an organization’s ability to derive actionable insights from big data."
In summary, the tools mentioned above—Apache Hadoop, Apache Spark, Tableau, and Microsoft Power BI—represent a selection of powerful solutions for big data analytics. Each tool offers unique advantages, making them suitable for various analytical needs and considerations. Businesses must assess their specific requirements and infrastructure to choose the best fit among these tools.
Case Studies: Success Stories
Case studies serve as crucial components in understanding the practical impact and the real-world applications of big data analytics software. They provide concrete examples that shed light on how organizations leverage data for improved outcomes. By examining these successes, decision-makers can gain insights into the effectiveness of different tools and approaches, ensuring they select the right solution tailored to their specific needs. Case studies also highlight challenges faced by organizations and how they overcame them, offering valuable lessons and best practices.
Retail Industry Applications
The retail sector is one of the primary beneficiaries of big data analytics. Retailers have access to vast amounts of data from customer transactions, online interactions, and supply chain activities. By adopting big data analytics software, they are able to personalize shopping experiences, optimize inventory management, and enhance marketing strategies.
For example, Target has successfully used data analytics to identify purchasing patterns and predict customer preferences. It can target promotions effectively, leading to increased sales and customer satisfaction. This approach not only boosts revenue but also strengthens customer loyalty, a vital aspect in a highly competitive marketplace.
Companies often utilize tools like Apache Hadoop and Tableau to analyze data streams from multiple sources.
- Personalized Marketing: Retailers are able to create targeted advertising campaigns based on individual shopping habits.
- Inventory Optimization: By analyzing sales trends, businesses can manage stock levels more efficiently, reducing waste and improving margins.
- Customer Insights: Data analytics helps retailers understand customer behavior, thus enabling better customer service.
Healthcare Sector Insights
In the healthcare industry, big data analytics plays a transformative role in improving patient care outcomes. Hospitals and health providers leverage analytics tools to sift through extensive patient records and treatment data. This capability allows them to identify trends and improve operational efficiency.
Organizations like Mount Sinai Health System have employed big data analytics to enhance patient care and streamline processes. By analyzing patient data, they have developed predictive models to recognize potential health risks, enabling earlier interventions that significantly improve patient health outcomes.
The following points highlight how analytics are utilized in healthcare:
- Predictive Modeling: This helps in anticipating patient admissions and optimizing resource allocation.
- Clinical Data Analysis: Aiding in identifying best practices and treatment protocols based on successful patient outcomes.
- Real-Time Monitoring: While utilizing analytics, healthcare providers can monitor patient vitals and alert for immediate interventions if deviations occur.
In summary, success stories in both the retail and healthcare sectors underscore the potential of big data analytics software. Organizations that harness this technology are positioned to achieve superior operational performance and enhanced customer satisfaction.
Challenges in Big Data Analytics Implementation
The implementation of big data analytics software is often marred by challenges that organizations must carefully navigate. Recognizing these challenges is paramount, as they can hinder the effective use of data within a company. By understanding the specific elements that contribute to these challenges, decision-makers can make better choices to enhance their data strategies and ensure successful implementations.
Data Quality Issues
Data quality is a foundational element that dictates the success of big data projects. Poor quality data can result in faulty analytics, leading to misguided business decisions. There are several factors contributing to data quality issues:
- Inaccurate Data: Incomplete or incorrect data can stem from various sources, including human error and outdated systems.
- Inconsistent Data: When data is collected from multiple sources, variations in formats and standards often arise.
- Outdated Information: Regular updates to data sources are crucial. Failing to do so can lead to analytics using stale data, resulting in insights that do not reflect current realities.
To combat these issues, organizations should prioritize data cleansing processes and implement robust data governance frameworks. A focus on proactive data management practices will lay a solid foundation for successful analytics.
Integration with Existing Systems
Another significant challenge lies in the integration of big data analytics software with existing systems. Many organizations face hurdles when attempting to align new analytical tools with legacy systems. Here are some considerations for addressing integration issues:
- Compatibility: Ensuring that the new software can seamlessly communicate with legacy systems is essential. Often, technical differences can cause roadblocks in data flow.
- Resource Allocation: Integrating new solutions may require substantial investment in terms of time, money, and personnel.
- Training: Employees need appropriate training to adapt to new tools. A lack of understanding can lead to underutilization of resources.
Integrating new big data solutions demands a well-structured plan. Decision-makers should be prepared to allocate necessary resources for training and adjustments to existing processes.
"Effective data integration leads to holistic insights, a critical component for informed decision making."
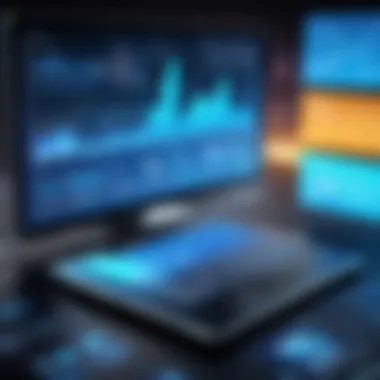
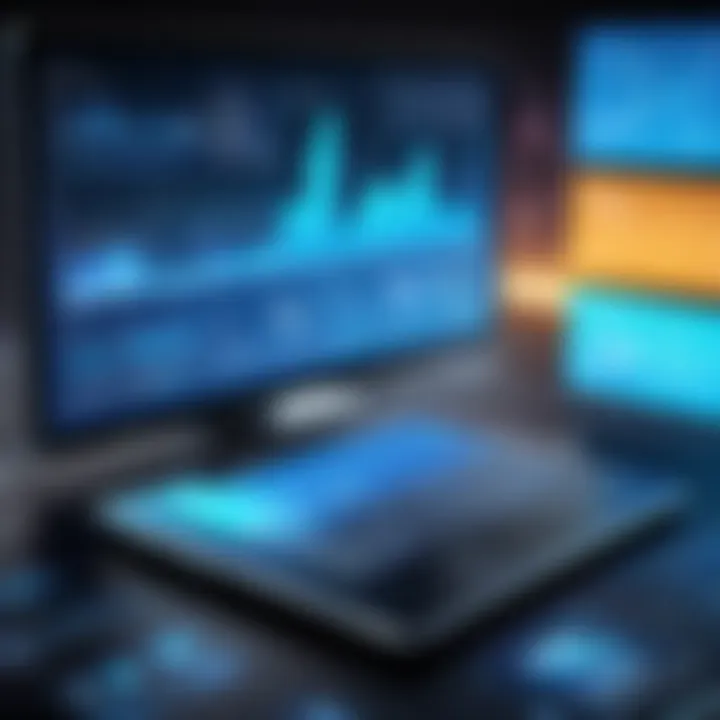
The challenges surrounding big data analytics implementation should not deter organizations. Instead, these challenges serve as guiding points for improvement. Acknowledging and addressing data quality and integration aspects will ultimately pave the way for successfully harnessing big data insights.
The Role of Artificial Intelligence in Big Data Analytics
Artificial Intelligence (AI) plays a pivotal role in the domain of big data analytics, significantly enhancing its capacity to extract actionable insights. In this age of information, where vast amounts of data are generated continuously, AI enables organizations to process and analyze this data efficiently. AI-powered analytics tools are designed to recognize patterns, trends, and anomalies within datasets, which can lead to better decision-making processes. As such, the interplay between AI and big data analytics shapes the future of business intelligence.
Enhancing Data Analysis
AI improves the quality and depth of data analysis in various ways. Machine learning algorithms, a subset of AI, can automatically learn from data without explicit programming. This means that as more data becomes available, these algorithms become more accurate and efficient in their predictions. For instance, companies can use predictive analytics to forecast sales trends, customer behaviors, and inventory needs based on historical data and real-time inputs.
Moreover, natural language processing (NLP) allows analysts to dig deeper into unstructured data, such as social media posts or customer reviews. By harnessing NLP, organizations can gauge public sentiment, derive consumer insights, and refine marketing strategies more effectively. AI's capability to synthesize diverse datasets enhances the overall efficacy of data analysis, making complex interpretations much simpler.
Automating Reporting Processes
The integration of AI in reporting processes is another vital benefit of adopting AI in big data analytics. Traditional reporting can be time-consuming and labor-intensive. However, with AI-driven tools, data can be transformed into visually appealing and comprehensible reports almost in real-time. Automation not only accelerates the reporting process but also minimizes the likelihood of human error.
Additionally, AI can generate insights in various formats, allowing stakeholders to receive customized reports tailored to their specific needs. This adaptability is invaluable for decision-makers who require quick access to relevant information. The result is a more agile business environment where organizations can respond promptly to changing market conditions and insights.
"AI is not just a tool; it transforms how we perceive and analyze our data, leading to strategic innovation."
Regulations and Compliance in Big Data Analytics
In the field of big data analytics, regulations and compliance are not mere formalities; they are critical components that shape data practices and organizational strategies. As businesses increasingly rely on data-driven insights, understanding and adhering to regulations is paramount. The significance of compliance can be seen across various dimensions, from avoiding legal penalties to fostering customer trust. Companies must navigate the complex landscape of laws and regulations that govern data collection, storage, and usage.
In many regions, data protection laws such as the General Data Protection Regulation (GDPR) in Europe are enforced strictly. These laws mandate how organizations handle personal data. Non-compliance with such regulations can lead to severe penalties, including hefty fines and reputational damage. Organizations need to prioritize compliance to ensure sustainable operations while minimizing risks associated with data breaches.
Data Privacy Concerns
Data privacy concerns are among the foremost issues in big data analytics. As organizations gather vast amounts of sensitive information, they must implement measures to protect this data from unauthorized access and misuse. Individuals are becoming more aware of how their data is used. Companies must be transparent about their data practices and articulate how they safeguard personal information.
Regulations often restrict how data can be collected and used. For instance, individuals have the right to know what data is collected about them and how it is processed. This requires organizations to maintain clear records of their data practices and provide users with accessible insights. Furthermore, companies may need to establish protocols for data deletion requests or provide options for data portability.
To address privacy concerns, organizations can consider the following actions:
- Establish clear data governance policies.
- Train employees on data privacy laws and ethical data handling.
- Utilize encryption and other security measures to protect sensitive data.
- Regularly audit data practices to identify and mitigate risks.
Compliance with Industry Standards
Compliance with industry standards goes beyond legal requirements; it encompasses best practices that enhance operational integrity. Industry standards, often established by regulatory bodies and industry groups, guide organizations on benchmarks for data handling and protection. Following these standards not only helps mitigate risks but also ensures consistency in data practices across the sector.
Standards such as the International Organization for Standardization (ISO) offer comprehensive frameworks that organizations can adopt. For example, ISO 27001 focuses on information security management. Organizations achieving certification demonstrate a commitment to data protection and risk management.
Moreover, compliance with industry standards often leads to improved operational efficiencies and stakeholder confidence. Maintaining industry standards allows for better collaboration within industry partners and fosters trust with clients.
In summary, navigating the landscape of regulations and compliance in big data analytics is a multi-faceted task. It requires a proactive approach to data privacy and strict adherence to industry standards. As the regulatory environment evolves, organizations must remain vigilant and adaptable to uphold their commitments to data protection and privacy.
Future Trends in Big Data Analytics
Understanding future trends in big data analytics is crucial for organizations aiming to stay competitive in a data-driven environment. As technology evolves, so do the strategies and tools used for data analysis. Big data analytics not only enhances insight generation but also drives decision-making processes that can significantly affect business outcomes. Organizations that recognize and adapt to these trends can leverage their data more effectively, providing them with a strategic edge.
Real-Time Analytics Advances
The demand for real-time analytics is growing as businesses require immediate insights to respond swiftly to market changes. Real-time processing enables organizations to analyze data continuously, rather than in batches. This means businesses can act on insights almost instantly, enhancing their agility and responsiveness. For example, retail companies can monitor customer behavior as it happens, allowing them to adjust marketing strategies or inventory levels dynamically.
In addition, advanced technologies, including Apache Kafka and Apache Flink, support real-time data streaming. They help in capturing and processing large amounts of data quickly. Companies utilizing these technologies often experience improved customer satisfaction and operational efficiency. The following are some key benefits of real-time analytics:
- Immediate decision-making: Organizations can make quick, informed decisions based on up-to-the-minute data.
- Increased operational efficiency: Continuous analysis of data can highlight inefficiencies and areas for improvement.
- Enhanced customer experiences: Businesses can tailor services to meet customer demands as they emerge.
Edge Computing Integration
The integration of edge computing with big data analytics is another trend that bears significance. Edge computing involves processing data near the source rather than relying solely on centralized data centers. This approach reduces latency and bandwidth use, which enhances the speed of data processing.
In sectors like manufacturing, edge computing allows for real-time data collection from IoT devices. Equipment can be monitored continuously, and any anomalies can be addressed promptly, reducing downtime and maintenance costs. The combination with big data analytics enables organizations to:
- Optimize performance: By processing data at the location of generation, systems can operate more efficiently.
- Enhance data security: Sensitive data can be processed locally, minimizing potential exposure during transmission.
- Scale operations more effectively: With capabilities to handle data on the edge, organizations can expand their operations without overloading central systems.
"The future of big data analytics will largely depend on the ability to process and analyze data at the edge, ensuring faster and more secure insights."
End
In this final section, we distill the integral points discussed throughout the article. Big data analytics software serves as a crucial tool for businesses navigating the complexities of large datasets. Understanding the functionalities and the benefits of utilizing such software can significantly enhance decision-making processes and drive innovation. Companies are increasingly relying on data to inform strategies and improve operational efficiency.
Summary of Key Insights
As we summarize the insights from this comprehensive exploration, several points stand out:
- Functionality: Big data analytics software is designed to handle vast amounts of data, enabling businesses to extract meaningful insights quickly and efficiently.
- Deployment Models: Various deployment options such as on-premises, cloud-based, and hybrid models cater to different business needs, providing flexibility in data management.
- Future Trends: The landscape of big data analytics is ever-evolving. Real-time analytics and edge computing integration are set to reshape how organizations utilize data.
- Challenges: Despite its potential, implementing big data analytics comes with challenges such as data quality and system integration. Addressing these requires careful planning and execution.
With an understanding of these elements, businesses can adapt their analytics strategies to stay competitive in an increasingly data-driven world.
Recommendations for Decision-Makers
For decision-makers looking to leverage big data analytics software effectively, the following recommendations can provide guidance:
- Assess Needs: Before selecting software, conduct a thorough assessment of your organization’s specific needs. Consider factors such as existing data infrastructure, budget limitations, and desired outcomes.
- Choose Vendor Wisely: Research vendors carefully. Look for those with reputable track records, positive user feedback, and robust customer support. Vendor reputation plays a vital role in the successful implementation of analytics solutions.
- Focus on Training: Invest in training for staff to ensure they can make the most of the analytics tools. This can lead to better adoption and ultimately improve the quality of insights derived from data.
- Stay Updated: Keep abreast of the latest developments in big data technologies. Being aware of innovations can position your organization to take advantage of emerging opportunities in data analytics.
By following these recommendations, organizations can effectively align their big data analytics initiatives with their broader strategic goals, maximizing the benefits of informed decision-making.